Welcome to the new frontier of technology: Generative Artificial Intelligence (AI). Generative AI is a subset of machine learning, a type of artificial intelligence that provides systems the ability to learn from data, where machines learn to generate data that is similar to the data they are trained on. This powerful tool allows AI to create new content, from writing complete sentences to developing unique designs, images, and music compositions, marking a paradigm shift in the AI landscape.
The game-changing potential of Generative AI lies in its capacity for creation and innovation. By providing machines with the ability to generate new data and content, we unlock previously unimaginable possibilities. Generative AI takes us beyond the realm of predictive and analytic capabilities, venturing into the uncharted territories of imagination and creativity. This is just the tip of the iceberg. As we dive deeper into the exploration and application of Generative AI, we set the stage for revolutionary breakthroughs in diverse sectors, from art and design to science and technology.
Overview of Generative AI
Historical Development of Generative AI
Generative AI has come a long way since its inception. The seeds of Generative AI were sown with the introduction of machine learning. As algorithms became more sophisticated, so did their capabilities. The development of Generative Adversarial Networks (GANs) by Ian Goodfellow and his colleagues in 2014 marked a significant milestone in the evolution of Generative AI, providing it with the capability to generate entirely new content.
Understanding How Generative AI Works
At its core, Generative AI works by learning the patterns and structures of the data it is trained on. It then uses this learned knowledge to generate new data that mirrors the structure and style of the input data. This is achieved through advanced machine learning models such as GANs and Variational Autoencoders (VAEs), which we will discuss further in this article.
Main Technologies Underpinning Generative AI: GANs, VAEs, etc.
Generative AI primarily relies on two types of machine learning models: Generative Adversarial Networks (GANs) and Variational Autoencoders (VAEs). GANs consist of two neural networks – the generator and the discriminator – that work together to generate new data. VAEs, on the other hand, are a type of autoencoder that adds a layer of complexity by introducing probabilistic rules, which enable them to generate a broader range of data.
Key Differences Between Generative AI and Discriminative AI
While both Generative and Discriminative models are part of machine learning, they serve different purposes. Discriminative models focus on distinguishing different types of data and are often used in classification problems. Generative models, on the other hand, learn the inherent distribution of the input data and can generate new data based on that distribution.
Breaking Down Generative Adversarial Networks (GANs)
Introduction to GANs
Generative Adversarial Networks (GANs) represent a leap forward in the world of Generative AI. They are a class of machine learning systems introduced by Ian Goodfellow and his colleagues in 2014. The power of GANs lies in their structure, which comprises two components that play a continuous game against each other: the Generator, which creates new data, and the Discriminator, which evaluates the generated data for authenticity.
How GANs Work: Generator vs Discriminator
GANs function through a dynamic interplay between their two components. The Generator creates new data, trying its best to produce data that resemble the real, training data. Meanwhile, the Discriminator evaluates the generated data, trying to distinguish between the real data and the data produced by the Generator. This interplay pushes the Generator to continuously improve its data generation process until the Discriminator can no longer distinguish between the generated data and the real data.
Exploring Real-World Applications of Generative AI
Use of Generative AI in Content Creation: Art, Music, Writing
One of the most captivating applications of Generative AI is in the realm of content creation, encompassing art, music, and writing. Generative AI has already been employed to create stunning artworks, compose intricate music pieces, and even generate human-like text. It offers creatives a unique tool to push the boundaries of their craft and bring novel ideas to life. For instance, OpenAI’s GPT-3 has been used to generate coherent, contextually relevant pieces of writing that are nearly indistinguishable from those written by humans.
Role of Generative AI in Scientific Research
In the world of scientific research, Generative AI has the potential to revolutionize processes and workflows. It can be utilized to generate synthetic data for research purposes, enable faster prototyping through the generation of 3D models, or aid in drug discovery by generating chemical compound structures. For example, the use of GANs in drug discovery has shown promise in creating new molecules with desired properties, accelerating the traditionally long process of drug development.
Generative AI’s Potential in Cybersecurity and Privacy
The potential of Generative AI also extends to the domain of cybersecurity and privacy. Generative AI can be used to create synthetic datasets that mimic the statistical properties of real datasets, without containing any actual sensitive information. This application is particularly relevant in an age where privacy concerns are paramount. Furthermore, Generative AI can be utilized in creating more robust security systems. For instance, GANs can be used to generate cyber-attack scenarios, allowing cybersecurity professionals to prepare more effectively.
The Future of Generative AI: Expert Predictions
As Generative AI continues to evolve, its potential applications will expand. Experts predict that Generative AI will play a pivotal role in numerous sectors, from fashion, where it could help design new clothing styles, to autonomous driving, where it could help in simulating various driving scenarios for training purposes. As we move towards this future, it’s important to prepare for the transformative impact of Generative AI.
Unpacking the Ethical and Regulatory Challenges of Generative AI
The Potential Misuse of Generative AI: Deepfakes
While the applications of Generative AI are impressive, they also open the door to potential misuse. One prominent example is the creation of deepfakes. Using GANs, bad actors can generate highly realistic but fake images or videos, which could be used to spread misinformation, cause harm to individuals, or even manipulate public opinion. This highlights the need for stringent measures to prevent the misuse of Generative AI technologies.
Ethical Concerns Surrounding Generative AI
Ethical considerations are paramount in the deployment of any AI technology, and Generative AI is no exception. One concern is the lack of transparency and interpretability in Generative AI models, which makes it difficult to understand how these models arrive at their outputs. This opacity raises questions about accountability and fairness. Moreover, there’s the issue of consent and ownership when it comes to generating data or content that mirrors or mimics real individuals or their work.
Regulatory Landscape of Generative AI
As with any fast-evolving technology, the regulatory landscape for Generative AI is still in its nascent stages. Policymakers are grappling with the challenges of creating regulations that protect individuals and societies from potential harm, while not stifling innovation. Ensuring ethical use of Generative AI, maintaining privacy, and addressing issues of accountability are some of the key aspects that need to be addressed in future regulations. The task at hand for regulators around the world is to strike a balance between these considerations, fostering an environment where Generative AI can be used responsibly and to its full potential.
Some FAQs Answered On The Relevant Topic
What is the difference between Generative AI and other AI techniques?
Generative AI is a subset of AI that focuses on creating new data models from existing data sets. Unlike discriminative AI, which classifies or predicts based on existing data, Generative AI can produce entirely new data. It does this through techniques like Generative Adversarial Networks (GANs), where a generator model creates new data and a discriminator model judges its quality.
How can Generative AI be applied in the industry?
Generative AI has a wide range of applications across different industries. In content creation, it can generate new music, images, or written content. In scientific research, it’s used for generating synthetic data or simulating scenarios for drug discovery. In cybersecurity, Generative AI can help create synthetic datasets for privacy or generate cyber-attack scenarios for better security planning. These are just a few examples, and the possibilities are growing with the technology.
What are the risks and ethical issues associated with Generative AI?
Like any technology, Generative AI comes with its own set of risks and ethical issues. The potential for misuse is significant, with deepfakes being a prominent example. These are realistic but fake images or videos created with GANs that can spread misinformation or cause harm. Ethically, there’s a need for transparency and accountability in Generative AI models, as well as consent and ownership issues when generating data or content that mimics real individuals or their work.
How can I get started with learning or developing Generative AI solutions?
Getting started with Generative AI requires a basic understanding of machine learning and AI principles. There are numerous online courses and resources that offer comprehensive introductions to Generative AI and its foundational techniques like GANs. Practical experience, such as working on projects or using Generative AI platforms, can also be beneficial.
In Conclusion, Generative AI represents a groundbreaking frontier in technology. It has the potential to revolutionize various sectors, from content creation and scientific research to cybersecurity and privacy. By producing new data from existing ones, it opens up a realm of possibilities that extend far beyond traditional AI techniques.
The Need for Continuous Education and Ethical Guidelines in the Development and Use of Generative AI
However, as with any powerful technology, it comes with significant ethical and regulatory challenges. It’s crucial that as we embrace the potential of Generative AI, we also maintain a strong focus on education and the development of ethical guidelines. This way, we can ensure that this technology is used responsibly and that its benefits are maximized for the greater good. As we continue to explore this exciting field, continuous learning and adaptation will be key to navigating its ever-evolving landscape.
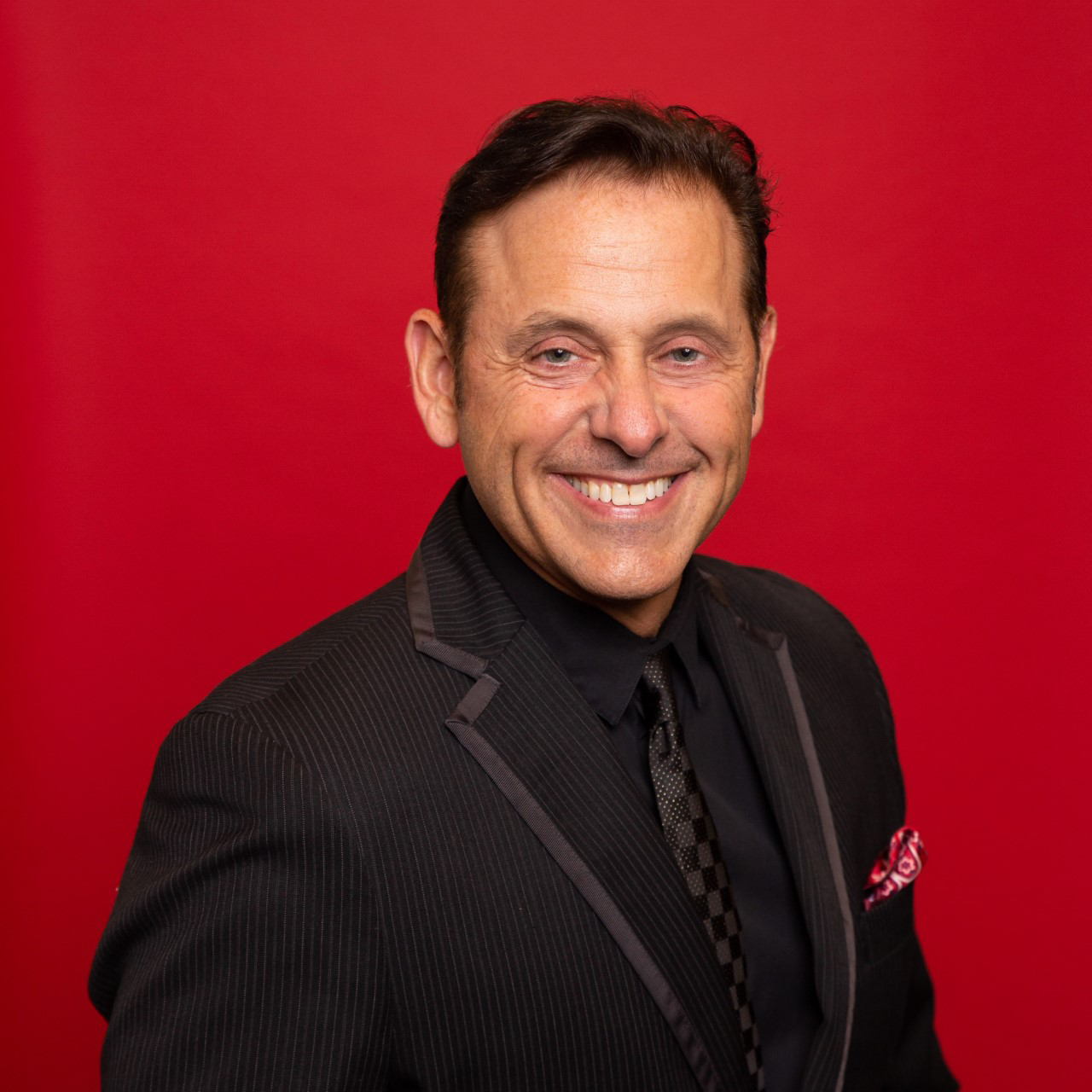
Eric Dalius is The Executive Chairman of MuzicSwipe, a music and content discovery platform designed to maximize artist discovery and optimize fan relationships. Outside of MuzicSwipe, he hosts the weekly podcast “FULLSPEED,” which highlights conversations with accomplished entrepreneurs. Eric is also committed to supporting education through the “Eric Dalius Foundation,” offering four scholarships to US students. Keep up with Eric on Twitter,YouTube, Facebook, LinkedIn, Instagram, and Entrepreneur.com.